This is the final instalment in a blog series authored by Napier about sanctions screening. So far, we have covered the key principles of sanctions screening, why financial organisations should be prioritising their AML due diligence, the current challenges with screening processes and legacy systems, and the best way to approach sanctions screening.
Introducing Napier’s screening software
Napier offers a complete and comprehensive customer, supplier, employee and transaction screening solution that combines the latest technology to minimise the incidence of false positives in the sanctions screening process by more than 90%.
Napier Client Screening can augment the capabilities of a legacy system by deploying its components on top of the existing system. This allows companies to significantly and cost-effectively improve the screening process in their current workflow with minimal system integration effort.
Napier implements a four-phase approach to enhance the efficiency and effectiveness of the screening process by optimising data context.
These phases are outlined below:
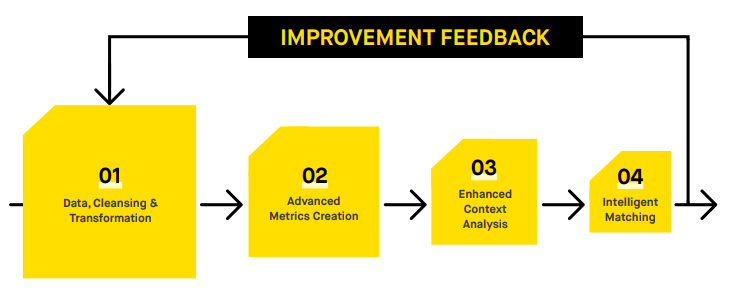
1. Data cleansing and transformation
Data stored in original data repositories, such as transactional systems and customer record systems, are ingested and processed by the system. This can be achieved using connects for specific systems, leveraging file transfers or by using an application programming interface (API). The system can also ingest already processed alerts and potential hits, aiming at using these as inputs in its false positive reduction process.
Once the data is ingested, it is then transformed and cleansed with initial filters applied based on the input type. For example, duplicates may be removed, and data may be enriched and normalised.
2. Advanced metrics creation
Metrics are generated based on data type. For example, a set of distance metrics is generated between the client's name and the client in a sanctions list. Initial thresholds are set based on current policies and risk appetite and can be configured by the client, such as acceptable spelling errors in a match. Features are then created to describe statistics of input data.
3. Enhanced context analysis
Input data is analysed in detail to weight terms based on context. For example, the engine assesses the weight of each term in a company name. In comparing the legal entities below, although the majority of the characters and words are similar, it is clear they are two different companies:
- Fortytwo Management Services and Partners
- Paper Management Services and Partners
Therefore, the context and relevance of each term is considered when performing a match.
4. Intelligent matching
Multiple techniques including policy application, rules and disparate scores are applied to the input data, leveraging initial thresholds and labels. The most relevant matches are presented for review with a detailed explanation for the reason the system is suggesting a review. The discounted entities are not presented to the user, however a detailed description explaining the reason for the dismissal is provided.
The Napier screening solution provides a natural language explanation for the reasons why matches have been discounted, including a link to policies and reference data used to automate the discount decision. This can be used to help justify the decision to the regulator, improve policies, and support data sampling.
Introducing Napier’s AI Advisor and additional functionality
Napier’s screening solution offers added functionality, including smarter false positive reduction with its unique AI Advisor feature:
Napier’s AI Advisor is an optional feature within Napier’s screening solutions that helps analysts review alerts faster, by identifying the false positives in screening.
By using machine learning to analyse screening outcomes and improve match scoring, it can determine if the match should be discounted or requires further review.
AI Advisor works alongside a rules-based approach: rules determine the screening matches, and AI Advisor determines how the good the match was, and if the match warrants further investigation. It does this by scoring each match, showing the components that contributed to the score in a clear visual on the screening dashboard to help analysts make quick decisions about the quality of the match.
AI Advisor also provides an explanation alongside the score to help analysts understand the key factors in its decision. This helps users understand why a match was created and what was unusual about it. The additional insights from AI Advisor, which analyses multiple additional variables to score a match, can help reduce false positives further by up to 40%.
Other features in Napier's screening solutions include:
Additional rule creation
Create additional rules to match specific scenarios, such as matching on nicknames, name variations and the use of phonetic variances for specific languages. Importantly, a trade-off has to be found between allowing for all name variations in all languages and focusing on the key ones.
Feature enrichment
Our solution adds features to the data to increase the likelihood of accurate matching. For example, by automatically deriving the gender of individuals from their given names using machine learning techniques. Another example is the use of machine learning to assess face similarity by comparing images gathered at onboarding with images from the sanction list provider.
Machine learning enabled
Napier's screening uses machine learning to improve matching scoring and implement champion versus challenger models.
Workflow
Napier's solution includes a workflow to continuously improve the screening process, by leveraging analyst feedback in automatically classifying matches as correct or incorrect.
Sanctions screening: how to reduce false positives in client and transaction screening
In this final instalment of our sanctions screening blog series, we delved further into how technology, like that of Napier, can revolutionise the sanctions screening process to minimise false results, help compliance officers and analysts work more efficiently, and protect organisations from costly penalties.
Napier’s screening solution uses the latest technology to help organisations prevent and fight money laundering and financial crime, and protect organisations from the repercussions of sanctions violations.
This article is the fifth in a series of a larger paper authored by Napier. If you would like to read the full paper, you can download it here.