As the Chief Data Scientist at Napier, I will uncover how AI can be used to analyse customer behaviour and identify emerging financial crime risks as a result of a worldwide pandemic.
No one could have predicted the difficulties we have all faced in the past years. COVID-19 has changed how we live and work. This change has been global and rapid, but crucially, it has also had a differing impact on various countries and industries.
Here I explore how to use AI to analyse customer behaviour and successfully identify emerging financial crime risks in what is a very testing environment.
Why is COVID-19 so challenging for AML?
There will always be natural market cycles of boom and bust but, in such cases, we have years of data available for comparative analysis. When a crash does happen, everyone is usually affected similarly and we can make fairly reliable predictions.
The problem with COVID-19 is that the financial crime patterns we are seeing this year are very different to the patterns we’ve seen in previous years. This can make it challenging to accurately identify abnormal and suspicious behaviour.
Moreover, we are seeing increased levels of coronavirus-related crime. COVID-19 business loan fraud, for example, is a big problem. At the same time, cash money laundering has become difficult owing to lockdown restrictions and rapidly increased use of cashless transactions, which means these launderers will be seeking other ways to wash their dirty money.
COVID-19 AML coping strategies
With the need to promptly and accurately identify financial crime more important than ever, there are three broad options for how you can apply AI to cope with the AML challenges this pandemic has presented:
1. Alternate universe
This rudimentary option acts as if COVID-19 hasn’t happened. You will simply use AI to predict where financial markets and companies would have been in a world without the virus, but with all other influences taken into consideration, ranging from politics to climate change.
This strategy is based on the premise that you try to identify unusual patterns in the future. But, as you might expect, this strategy has a lot of weaknesses; not least because this isn’t what actually happened. Moreover, industries may not have recovered to where they were in 2018/19 let alone matched any predicted growth into the next year.
2. Monitor data and retrain AI
This would be the most popular strategy. Rather than ignoring the pandemic, this approach focuses on monitoring data and retraining AI based on the data that comes in and waiting for the models to adapt to the changing data.
COVID-19 is causing global disruption with micro consequences. Some businesses adapted quickly and changed their business model really well. Many are thriving. Others sadly closed down months ago. The severe changes we’ve seen mean predictions will be affected so the accuracy in identifying anomalies will drop in the short term.
3. Split data by industry
Crucially, COVID-19 has and continues to affect industries differently. This strategy recognises that you can’t pour all your data into one pot, give it a stir and hope AI makes good of it. Data must be processed in a much more analytical way.
Splitting data by specific industries is a sophisticated, best practice technique. Compared to the other strategies it is more complex, requiring greater effort and data understanding, including the introduction of several analysis models (see below). The payoff however is high: easy and accurate identification of anomalies, such as suspiciously high growth.
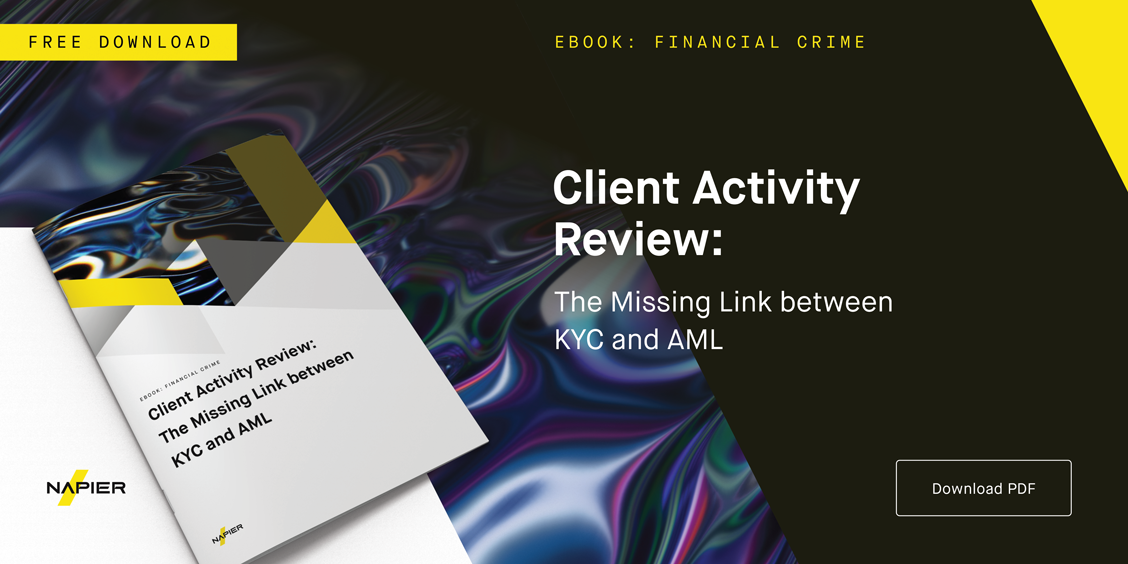
Client Activity Review: The Missing Link Between AML and KYC.
The need for a modular approach that complements rule-based monitoring
A modular approach that complements rule-based monitoring is integral to the successful identification of anomalous transactions.
I strongly recommend adopting the creation of three different types of AI models:
1.) Local model – comparing the customer with itself over specified time periods and looking for changes in their specific activity.
2.) Global model – considering if the customer’s behaviour is unusual compared to everyone else.
3.) Segmented model – considering if the customer’s behaviour is unusual compared to people of a similar profile, such as that based on demography, industry, size, etc.
This modular approach ensures rules can quickly pick up patterns and behaviours you know about, while AI is able to thoroughly and accurately pick up the unknown unknowns. Combined together, it is very powerful.
Requirements and recommendations for the effective use of AI to monitor customer behaviour
The biggest problem I see is out-of-date systems.
Industry changes so quickly in terms of what’s suspicious and what’s unusual, as criminals attempt to circumvent detection, that it’s very hard to create a set of rules that will accurately find all of the suspicious transactions and customers.
Similarly, if an AI system can’t be updated easily, then it’s not going to pick up changing patterns. This is real challenge for the effectiveness of AML strategies. The system works fine to start with but overtime the performance significantly deteriorates – a bit like a car with no maintenance.
My recommendations for the effective use of AI to monitor customer behaviour are as follows:
Constantly test and update
Your AI system must be constantly learning so it is ready to spot new patterns. I recommend retraining AI as soon as new data comes in, so you’re always able to look for new differences.
Bad AI systems are ones that have been trained but rarely get retrained or updated. Data is trained in one scenario but over time the real world changes and the model doesn’t change. It means that what the system is predicting is no longer appropriate. This phenomenon is known as model drift. For example, we wouldn’t predict the snowfall for winter 2021 based on a model built in the 80s – that would be completely irrelevant now.
Consider how you will train AI carefully
One way of training and updating AI systems is through supervised learning – where humans identify unusual patterns as part of the machine’s training. But this is quite a time consuming task requiring so much effort that it often doesn’t get done.
At Napier, we use unsupervised learning because we are simply asking the system to look for patterns in data to identify any differences that are not already on the radar. These unknown unknowns can then be highlighted and investigated. This is an effective but low maintenance option and ensures AI training isn’t neglected.
Develop comprehensive datasets and rules
AI needs and works on comprehensive datasets with regular testing and training. As new things are discovered, it is important to add new rules as these can pick things up really quickly. This would mean slightly stricter rules. It is also really important to know your customer and maintain basic financial hygiene.
Adopt a modular approach to monitor customer behaviour
Finally, as I described above, you must avoid throwing all your data into one bucket. The three-part modular approach I described above, combined with rules, is essential.
Discover the optimal path to implement AI into your AML programme in this interactive webinar hosted in collaboration with Fintrail.
Find out how AI can improve your compliance processes
For information, advice or to book a demo on any Napier product, please get in touch with our expert team.