In our second webinar on financial crime typologies organised in partnership with RegTech Associates, we put together an expert panel, including Napier’s Chief Data Scientist, Dr Janet Bastiman. During the session, the panel explored the need for collaboration on the development and sharing of financial crime typologies, and how artificial intelligence can help to support and enhance this type of collaboration.
The webinar was moderated by Dr Sian Lewin, Co-Founder & Head of Client Delivery at RegTech Associates, and included:
- Nick Lewis, OBE - Managing Director, Conduct, Financial Crime and Compliance, Standard Chartered Bank
- Denisse Rudich, CCO & Co-Founder, ElementaryB and Director, Rudich Advisory
- Dr Janet Bastiman – Chief Data Scientist, Napier
You can watch the event replay below, or keep reading for a recap of the key talking points and conclusions of the panel.
To set the scene, Nick Lewis explained what financial crime typologies are, how they are developed, and what some of their shortcomings are.
Financial crime typologies
Nick highlighted that ‘financial crime typologies are misunderstood.’
Typologies are the categories of money-laundering red flags, often issued by standard-setting bodies such as the Financial Action Task Force (FATF) and national Financial Intelligence Units such as the UK’s National Crime Agency.
They are used by financial institutions to identify patterns in transaction data and customer behaviour that may indicate suspicious or illicit activities associated with specific types of financial crimes.
The creation of these typologies is a largely manual process, updates are infrequent, and they are always predicted on past behaviour.
It can also be difficult to get agreement on typologies owing to different legal systems and definitions about what constitutes a red flag in various jurisdictions.
However, collaboration through firms sharing information highlights a significant opportunity for combating financial crime, as firms can gain a fuller view of the patterns and methods used by criminals to launder money.
Nick said ‘there are huge opportunities for collaboration in both understanding methodologies and how they apply to different institutions and clients.’ He also pointed out that, ‘where technology develops, being able to share the data and metadata behind those patterns of behaviour across the institutions in a way that will increase our knowledge of typologies will result in increased protection.’
Over the course of the session, the panel discussed how AI can be influential in enabling collaboration in the anti-financial crime space.
How AI can help to identify financial crime typologies
Dr. Janet Bastiman gave an overview of the effectiveness of AI in tackling financial crime, and argued that one of AI’s most useful applications in an anti-money laundering (AML) context is ‘identifying things that are the same and things that are different and unusual.’
Applying AI to transactions and client activity means you can build up a pattern of ‘normal’ behaviour for a client from which the AI system can then detect and highlight outliers.
AI also has the advantage of operating at a level of speed and accuracy that is just not possible for humans to achieve.
Janet explained that ‘given the nature of financial crime, criminals try to blend in with genuine customers and transactions. AI is very good at detecting who a criminal is - for the criminal to get money into a system something very unusual needs to be done.
AI is extremely effective in highlighting these anomalous data points; AI’s inherent fuzziness detects these occurrences even when an exact match is absent. Janet explained that when criminals use similar methods to launder their money, because these patterns are known, AI is very good at uncovering them through supervised machine learning.
Unknown unknowns in AI Where things get more difficult is identifying the ‘unknown unknowns’, the emerging typologies which are the hardest to find and which target the gaps between existing rules and AI systems. However, unsupervised machine learning can be a very powerful tool in these situations.
Janet neatly summarised that ‘to find the unknown you either must remove the hay [the ‘normal’ data] or make the needle bigger [highlight the unusual].’
And this is exactly what unsupervised machine learning models do.
Importantly, Janet noted that, with predicate crimes, criminals continuously try new and different techniques to make their money look legitimate. This disrupts the ‘learnt’ patterns making it difficult to identify typologies and making detection through AI more challenging unless you are using time-sensitive models and developing new or tuning existing models in parallel.
Information sharing partnerships work
Collaboration is occurring effectively in some of the existing information sharing partnerships. Denisse Rudich explained that groups and forums such as the UK’s Joint Money Laundering Intelligence Taskforce and Australia’s Fintel Alliance are proving to be successful in the sharing of patterns of criminal behaviour and intelligence around financial crime.
These forums bring together actors from law enforcement, financial institutions, and NGOs, all of whom have access to different types of data – the combining of which is necessary to get a complete picture of money laundering typologies.
AI’s role in public-private relationships
Denisse highlighted areas where further development is necessary. Many of the existing public-private information sharing partnerships operate within borders but improvements need to be made in sharing information across borders.
One example is the need for a greater exchange of information between the UN (United Nations) and the Criminal Court of Justice to improve the effectiveness of financial crime fighting. Janet mentioned the potential role of AI through the collaboration between models, referencing GPT3, an unbiased network machine learning model, which enables the sharing of AI models. A similar network could be applied to the financial services sector.
Overcoming barriers to collaboration
All panellists agreed that the biggest obstacle to overcome in collaborating and sharing typologies is the conflict between information sharing and compliance with privacy laws such as GDPR.
Under these types of regulation, criminals are afforded the same levels of data privacy and protection as everyone else.
Fortunately, however, when it comes to typologies, the underlying data with personal identifiable information does not need to be shared. Typologies are more concerned with patterns of behaviour and the techniques used by criminals to exploit financial products and services rather than the specific activity of a specific person.
Under existing global FATF recommendations, financial institutions are under no regulatory obligation to share information or identify links between typologies and predicate crimes. Therefore, one of the biggest catalysts for greater information sharing would be for international standard setters to mandate collaboration. This would give a broader template for governments and institutions to follow, thus helping with financial crime issues which the private sector cannot fix alone.
Supported by innovative technologies such as AI, identifying emerging threats and risk indicators can be achieved more quickly through enhanced level of collaboration.
Be the first to know about Napier’s events
Sign up to our latest industry insights to be the first to know what’s going on in and around the world of Napier and compliance. Or follow us on LinkedIn and Twitter.
Photo by Joakim Honkasalo on Unsplash
Related content:
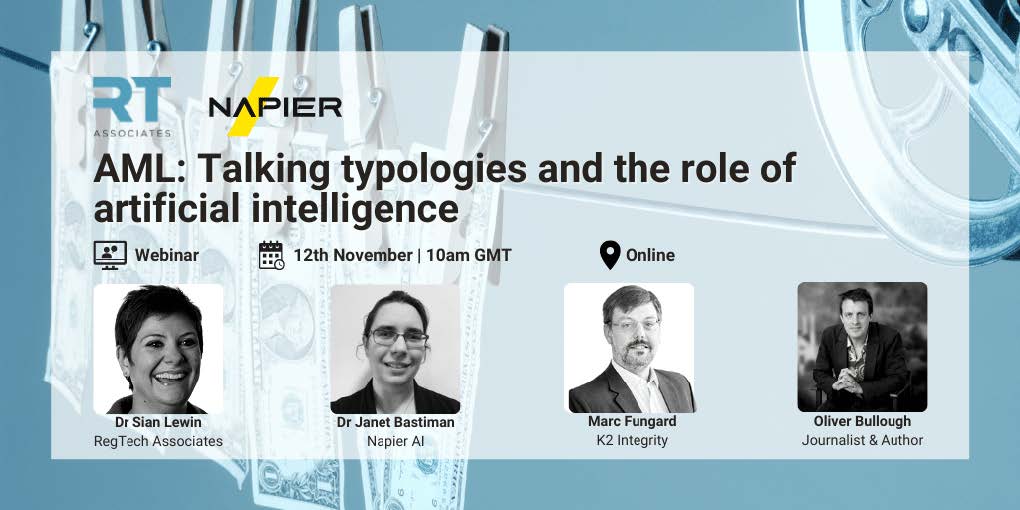